Empirical Evaluation of SNN for IoT Network Anomaly Detection
Vol. 49, No. 11, pp. 1497-1509, Nov. 2024

PDF Full-Text
Abstract
Statistics
Cumulative Counts from November, 2022
Multiple requests among the same browser session are counted as one view. If you mouse over a chart, the values of data points will be shown.
Multiple requests among the same browser session are counted as one view. If you mouse over a chart, the values of data points will be shown.
|
Cite this article
[IEEE Style]
Y. Lim, "Empirical Evaluation of SNN for IoT Network Anomaly Detection," The Journal of Korean Institute of Communications and Information Sciences, vol. 49, no. 11, pp. 1497-1509, 2024. DOI: 10.7840/kics.2024.49.11.1497.
[ACM Style]
Yeon-sup Lim. 2024. Empirical Evaluation of SNN for IoT Network Anomaly Detection. The Journal of Korean Institute of Communications and Information Sciences, 49, 11, (2024), 1497-1509. DOI: 10.7840/kics.2024.49.11.1497.
[KICS Style]
Yeon-sup Lim, "Empirical Evaluation of SNN for IoT Network Anomaly Detection," The Journal of Korean Institute of Communications and Information Sciences, vol. 49, no. 11, pp. 1497-1509, 11. 2024. (https://doi.org/10.7840/kics.2024.49.11.1497)
Vol. 49, No. 11 Index
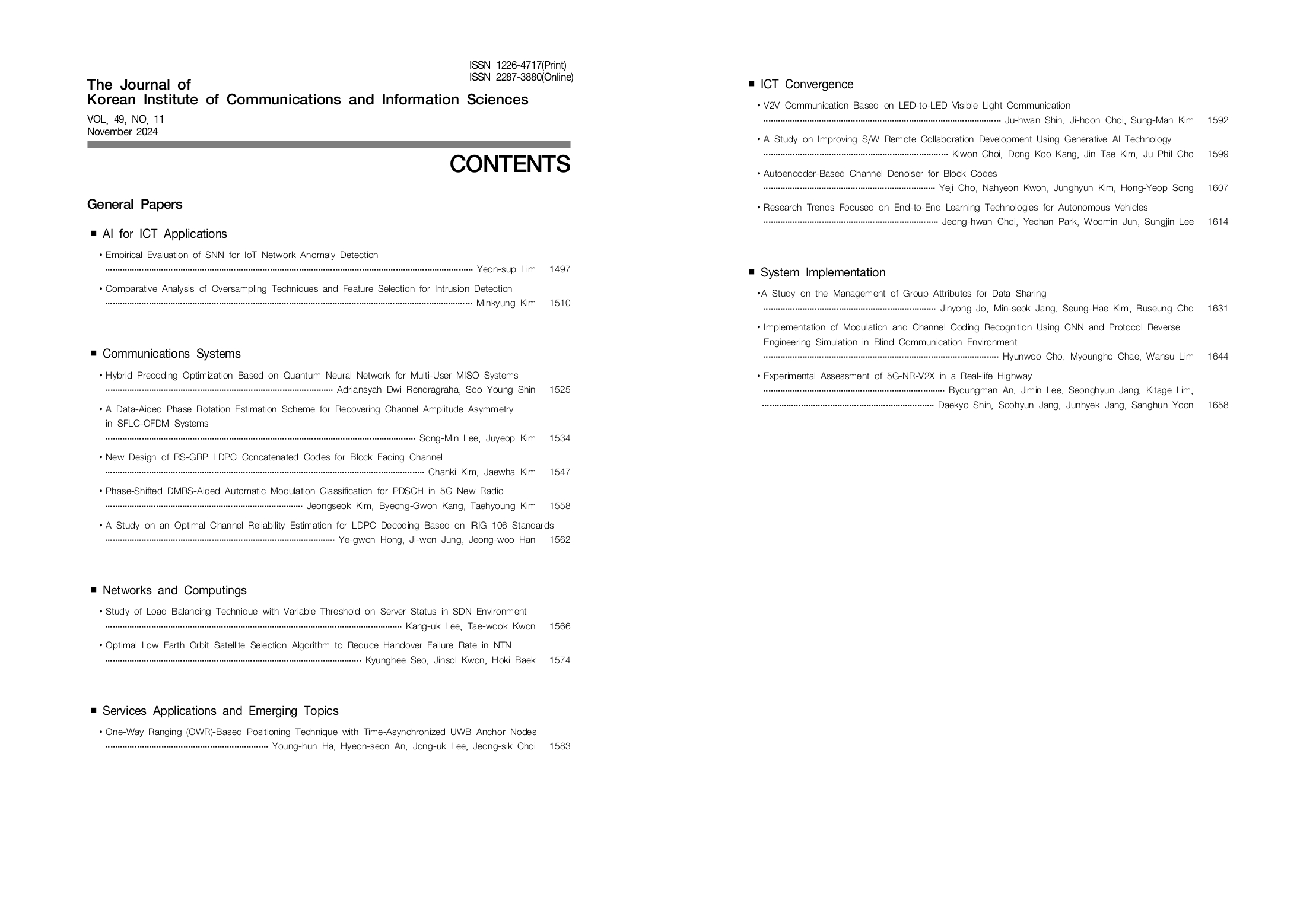