Energy-Efficient Artificial Neural Network Inference Based on Neural Network Partitioning
Vol. 48, No. 4, pp. 405-408, Apr. 2023

PDF Full-Text
Abstract
Statistics
Cumulative Counts from November, 2022
Multiple requests among the same browser session are counted as one view. If you mouse over a chart, the values of data points will be shown.
Multiple requests among the same browser session are counted as one view. If you mouse over a chart, the values of data points will be shown.
|
Cite this article
[IEEE Style]
SangseokYun, "Energy-Efficient Artificial Neural Network Inference Based on Neural Network Partitioning," The Journal of Korean Institute of Communications and Information Sciences, vol. 48, no. 4, pp. 405-408, 2023. DOI: 10.7840/kics.2023.48.4.405.
[ACM Style]
SangseokYun. 2023. Energy-Efficient Artificial Neural Network Inference Based on Neural Network Partitioning. The Journal of Korean Institute of Communications and Information Sciences, 48, 4, (2023), 405-408. DOI: 10.7840/kics.2023.48.4.405.
[KICS Style]
SangseokYun, "Energy-Efficient Artificial Neural Network Inference Based on Neural Network Partitioning," The Journal of Korean Institute of Communications and Information Sciences, vol. 48, no. 4, pp. 405-408, 4. 2023. (https://doi.org/10.7840/kics.2023.48.4.405)
Vol. 48, No. 4 Index
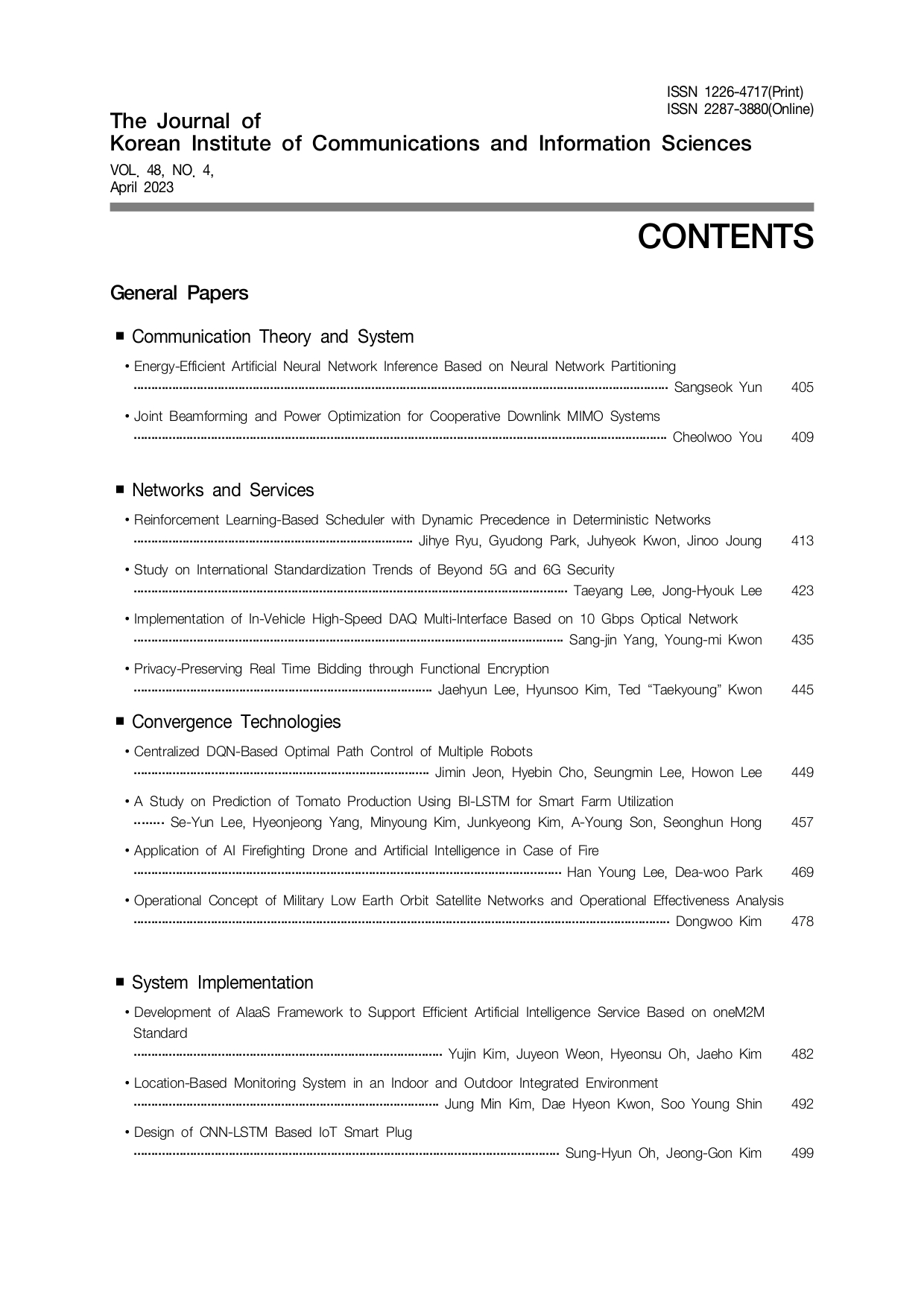