A New Energy-Based Latent-Variable Model for Unsupervised Feature Learning
Vol. 48, No. 5, pp. 509-516, May 2023

-
unsupervised feature learning latent variable model energy-based model Restricted Boltzmann Machine Image Classification
Abstract
Statistics
Cumulative Counts from November, 2022
Multiple requests among the same browser session are counted as one view. If you mouse over a chart, the values of data points will be shown.
Multiple requests among the same browser session are counted as one view. If you mouse over a chart, the values of data points will be shown.
|
Cite this article
[IEEE Style]
GuoPeng and DongKookKim, "A New Energy-Based Latent-Variable Model for Unsupervised Feature Learning," The Journal of Korean Institute of Communications and Information Sciences, vol. 48, no. 5, pp. 509-516, 2023. DOI: 10.7840/kics.2023.48.5.509.
[ACM Style]
GuoPeng and DongKookKim. 2023. A New Energy-Based Latent-Variable Model for Unsupervised Feature Learning. The Journal of Korean Institute of Communications and Information Sciences, 48, 5, (2023), 509-516. DOI: 10.7840/kics.2023.48.5.509.
[KICS Style]
GuoPeng and DongKookKim, "A New Energy-Based Latent-Variable Model for Unsupervised Feature Learning," The Journal of Korean Institute of Communications and Information Sciences, vol. 48, no. 5, pp. 509-516, 5. 2023. (https://doi.org/10.7840/kics.2023.48.5.509)
Vol. 48, No. 5 Index
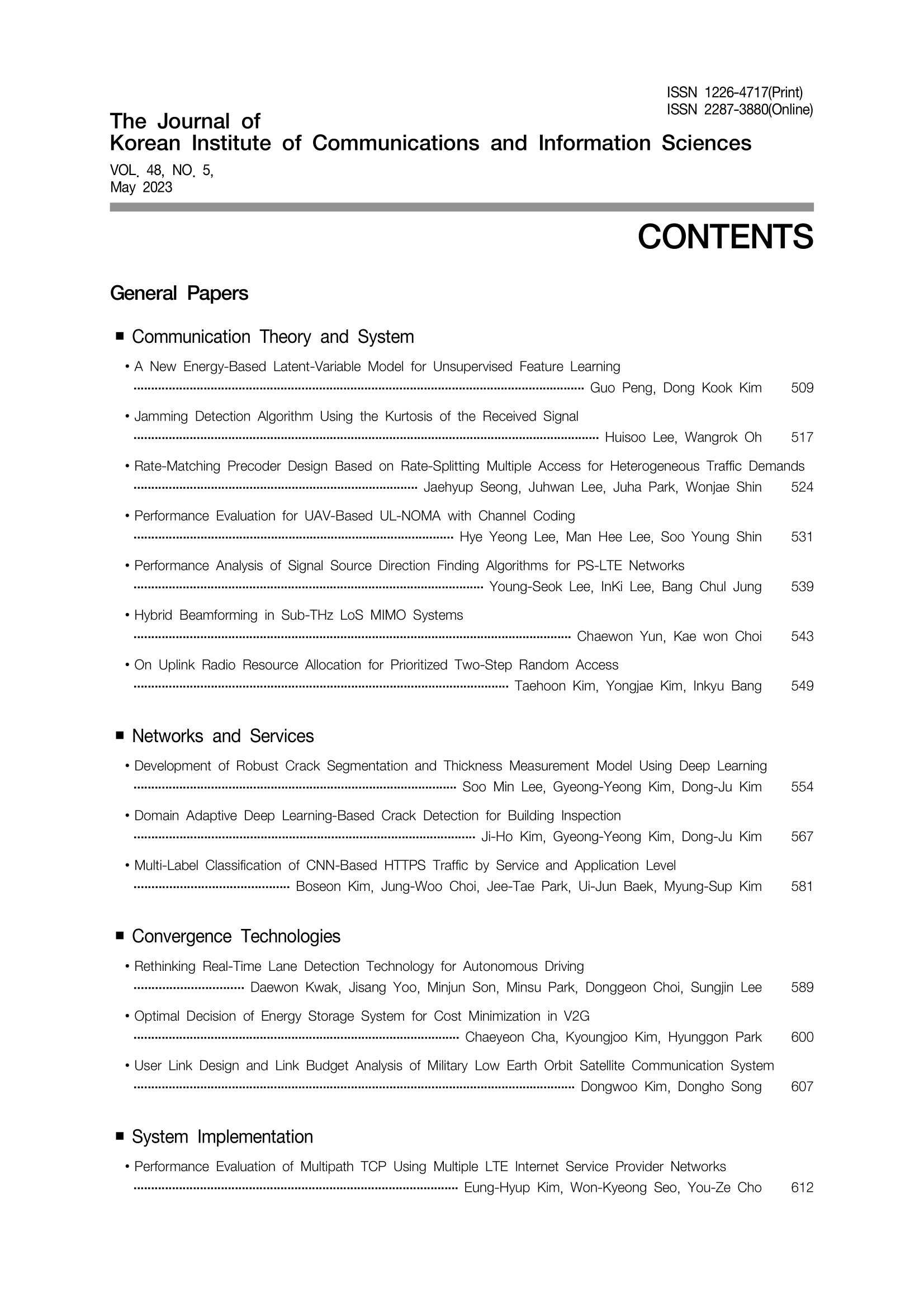