Development of Robust Crack Segmentation and Thickness Measurement Model Using Deep Learning
Vol. 48, No. 5, pp. 554-566, May 2023

Abstract
Statistics
Cumulative Counts from November, 2022
Multiple requests among the same browser session are counted as one view. If you mouse over a chart, the values of data points will be shown.
Multiple requests among the same browser session are counted as one view. If you mouse over a chart, the values of data points will be shown.
|
Cite this article
[IEEE Style]
SooMinLee, Gyeong-YeongKim, Dong-JuKim, "Development of Robust Crack Segmentation and Thickness Measurement Model Using Deep Learning," The Journal of Korean Institute of Communications and Information Sciences, vol. 48, no. 5, pp. 554-566, 2023. DOI: 10.7840/kics.2023.48.5.554.
[ACM Style]
SooMinLee, Gyeong-YeongKim, and Dong-JuKim. 2023. Development of Robust Crack Segmentation and Thickness Measurement Model Using Deep Learning. The Journal of Korean Institute of Communications and Information Sciences, 48, 5, (2023), 554-566. DOI: 10.7840/kics.2023.48.5.554.
[KICS Style]
SooMinLee, Gyeong-YeongKim, Dong-JuKim, "Development of Robust Crack Segmentation and Thickness Measurement Model Using Deep Learning," The Journal of Korean Institute of Communications and Information Sciences, vol. 48, no. 5, pp. 554-566, 5. 2023. (https://doi.org/10.7840/kics.2023.48.5.554)
Vol. 48, No. 5 Index
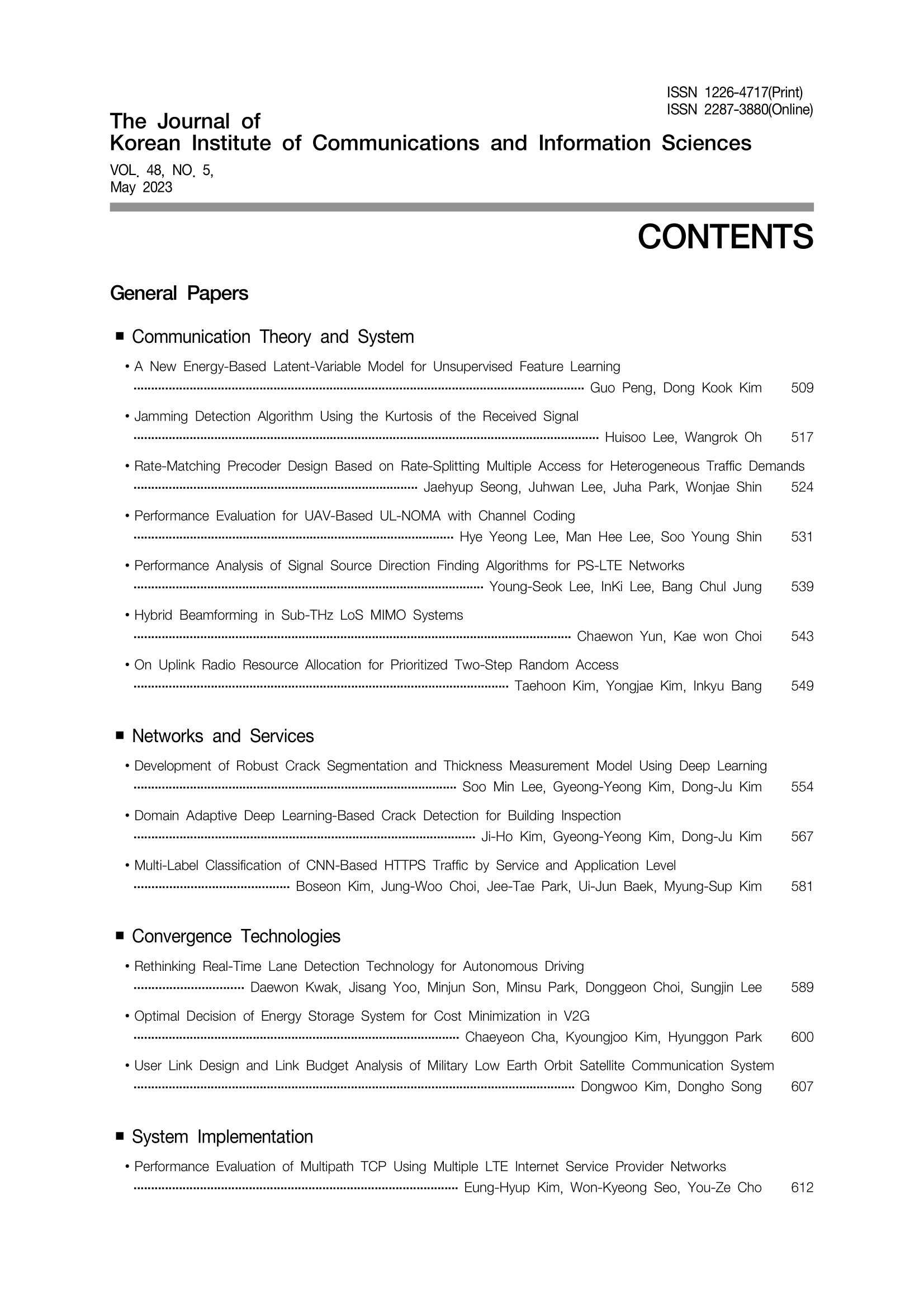