Stock Price Prediction Methodology Using Shallow Convolutional Neural Network
Vol. 48, No. 6, pp. 751-754, Jun. 2023

PDF Full-Text
Abstract
Statistics
Cumulative Counts from November, 2022
Multiple requests among the same browser session are counted as one view. If you mouse over a chart, the values of data points will be shown.
Multiple requests among the same browser session are counted as one view. If you mouse over a chart, the values of data points will be shown.
|
Cite this article
[IEEE Style]
Y. Cho, E. Kim, H. Shin, Y. Choi, "Stock Price Prediction Methodology Using Shallow Convolutional Neural Network," The Journal of Korean Institute of Communications and Information Sciences, vol. 48, no. 6, pp. 751-754, 2023. DOI: 10.7840/kics.2023.48.6.751.
[ACM Style]
Young-Jin Cho, Eui-Yeon Kim, Hong-Gi Shin, and Yong-Hoon Choi. 2023. Stock Price Prediction Methodology Using Shallow Convolutional Neural Network. The Journal of Korean Institute of Communications and Information Sciences, 48, 6, (2023), 751-754. DOI: 10.7840/kics.2023.48.6.751.
[KICS Style]
Young-Jin Cho, Eui-Yeon Kim, Hong-Gi Shin, Yong-Hoon Choi, "Stock Price Prediction Methodology Using Shallow Convolutional Neural Network," The Journal of Korean Institute of Communications and Information Sciences, vol. 48, no. 6, pp. 751-754, 6. 2023. (https://doi.org/10.7840/kics.2023.48.6.751)
Vol. 48, No. 6 Index
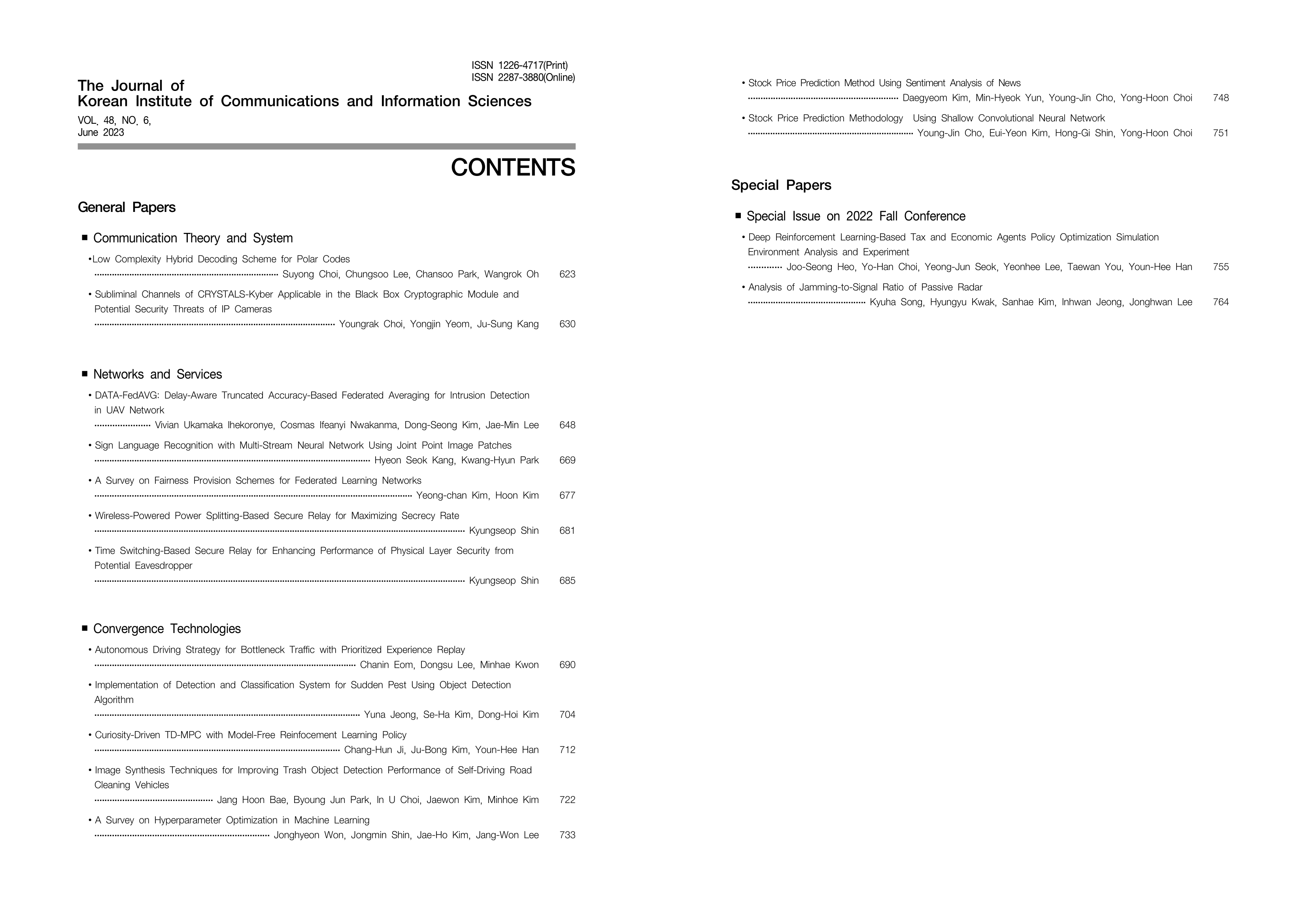