Semantic Segmentation of Satellite Images Based on an Improved U-Net Model with Dual Inputs
Vol. 48, No. 10, pp. 1219-1222, Oct. 2023

Abstract
Statistics
Cumulative Counts from November, 2022
Multiple requests among the same browser session are counted as one view. If you mouse over a chart, the values of data points will be shown.
Multiple requests among the same browser session are counted as one view. If you mouse over a chart, the values of data points will be shown.
|
Cite this article
[IEEE Style]
Y. Lee, J. Jung, Y. Shin, "Semantic Segmentation of Satellite Images Based on an Improved U-Net Model with Dual Inputs," The Journal of Korean Institute of Communications and Information Sciences, vol. 48, no. 10, pp. 1219-1222, 2023. DOI: 10.7840/kics.2023.48.10.1219.
[ACM Style]
Yelim Lee, Jin-won Jung, and Yoan Shin. 2023. Semantic Segmentation of Satellite Images Based on an Improved U-Net Model with Dual Inputs. The Journal of Korean Institute of Communications and Information Sciences, 48, 10, (2023), 1219-1222. DOI: 10.7840/kics.2023.48.10.1219.
[KICS Style]
Yelim Lee, Jin-won Jung, Yoan Shin, "Semantic Segmentation of Satellite Images Based on an Improved U-Net Model with Dual Inputs," The Journal of Korean Institute of Communications and Information Sciences, vol. 48, no. 10, pp. 1219-1222, 10. 2023. (https://doi.org/10.7840/kics.2023.48.10.1219)
Vol. 48, No. 10 Index
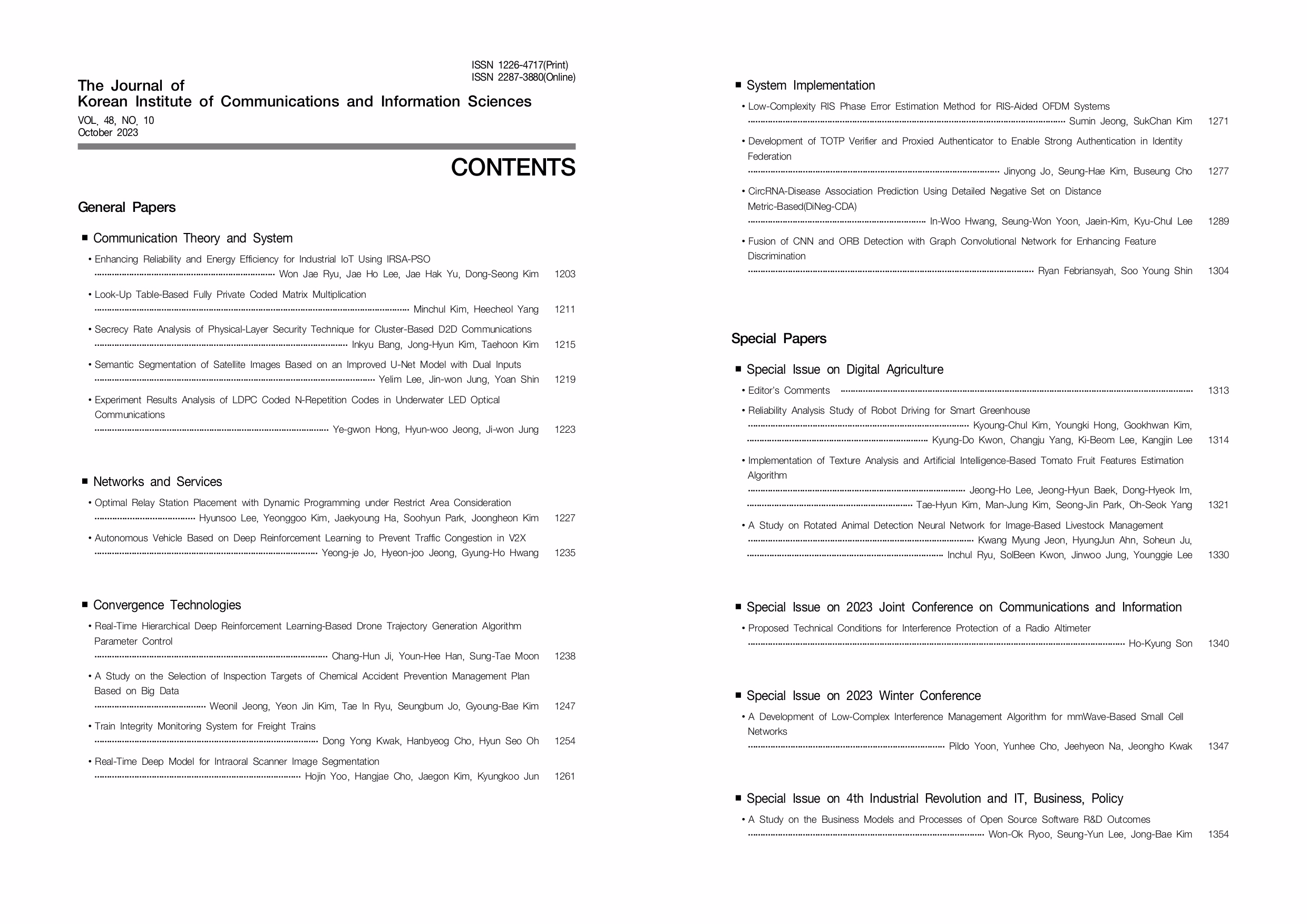