Best Papers Fine-Tuning Anomaly Classifier for Unbalanced Network Data
Vol. 49, No. 7, pp. 911-922, Jul. 2024

PDF Full-Text
Abstract
Statistics
Cumulative Counts from November, 2022
Multiple requests among the same browser session are counted as one view. If you mouse over a chart, the values of data points will be shown.
Multiple requests among the same browser session are counted as one view. If you mouse over a chart, the values of data points will be shown.
|
Cite this article
[IEEE Style]
M. Joe, M. Kim, M. Kwon, "Fine-Tuning Anomaly Classifier for Unbalanced Network Data," The Journal of Korean Institute of Communications and Information Sciences, vol. 49, no. 7, pp. 911-922, 2024. DOI: 10.7840/kics.2024.49.7.911.
[ACM Style]
Mugon Joe, Miru Kim, and Minhae Kwon. 2024. Fine-Tuning Anomaly Classifier for Unbalanced Network Data. The Journal of Korean Institute of Communications and Information Sciences, 49, 7, (2024), 911-922. DOI: 10.7840/kics.2024.49.7.911.
[KICS Style]
Mugon Joe, Miru Kim, Minhae Kwon, "Fine-Tuning Anomaly Classifier for Unbalanced Network Data," The Journal of Korean Institute of Communications and Information Sciences, vol. 49, no. 7, pp. 911-922, 7. 2024. (https://doi.org/10.7840/kics.2024.49.7.911)
Vol. 49, No. 7 Index
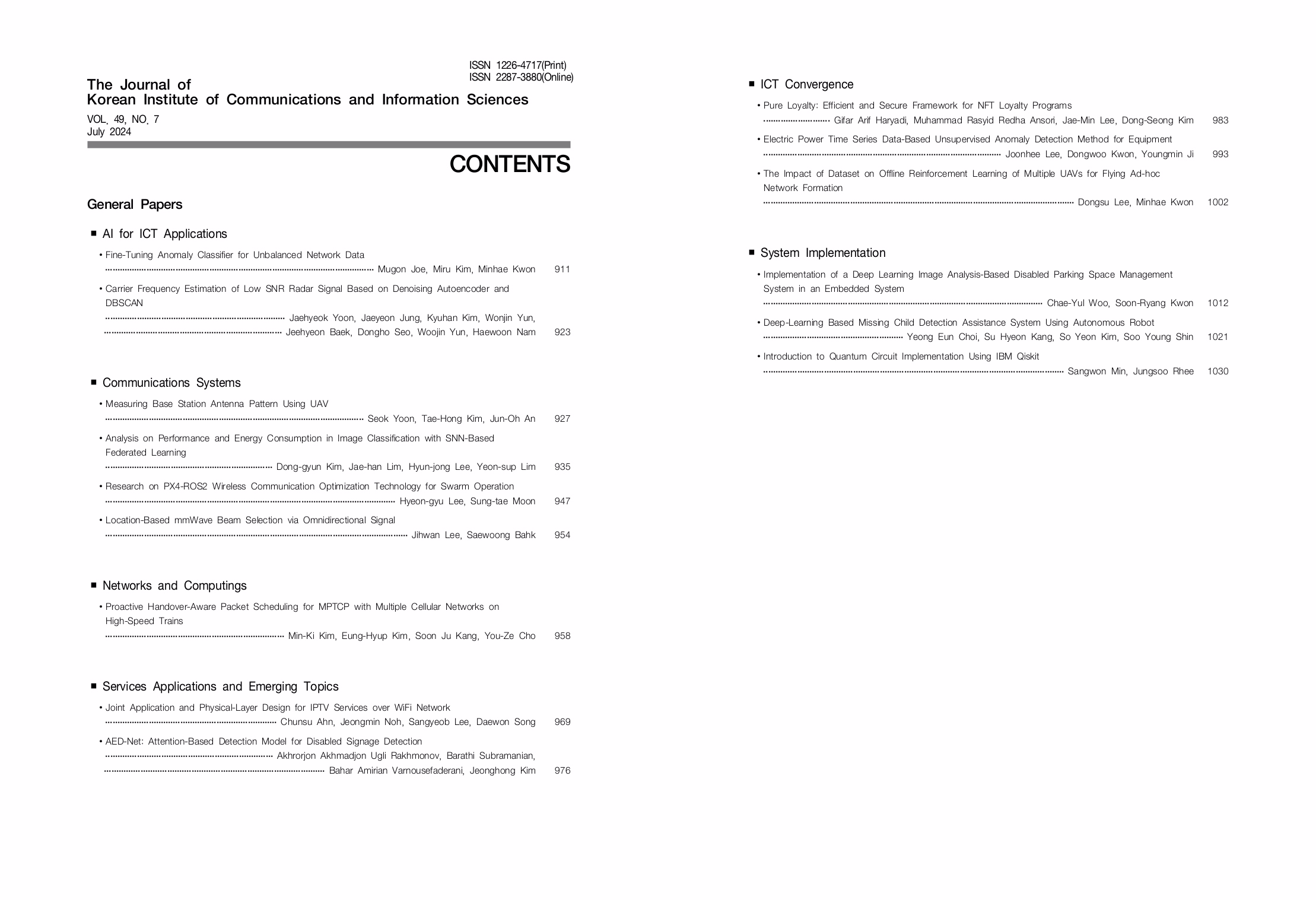