AED-Net: Attention-Based Detection Model for Disabled Signage Detection
Vol. 49, No. 7, pp. 976-982, Jul. 2024

PDF Full-Text
Abstract
Statistics
Cumulative Counts from November, 2022
Multiple requests among the same browser session are counted as one view. If you mouse over a chart, the values of data points will be shown.
Multiple requests among the same browser session are counted as one view. If you mouse over a chart, the values of data points will be shown.
|
Cite this article
[IEEE Style]
A. A. U. Rakhmonov, B. Subramanian, B. A. Varnousefaderani, J. Kim, "AED-Net: Attention-Based Detection Model for Disabled Signage Detection," The Journal of Korean Institute of Communications and Information Sciences, vol. 49, no. 7, pp. 976-982, 2024. DOI: 10.7840/kics.2024.49.7.976.
[ACM Style]
Akhrorjon Akhmadjon Ugli Rakhmonov, Barathi Subramanian, Bahar Amirian Varnousefaderani, and Jeonghong Kim. 2024. AED-Net: Attention-Based Detection Model for Disabled Signage Detection. The Journal of Korean Institute of Communications and Information Sciences, 49, 7, (2024), 976-982. DOI: 10.7840/kics.2024.49.7.976.
[KICS Style]
Akhrorjon Akhmadjon Ugli Rakhmonov, Barathi Subramanian, Bahar Amirian Varnousefaderani, Jeonghong Kim, "AED-Net: Attention-Based Detection Model for Disabled Signage Detection," The Journal of Korean Institute of Communications and Information Sciences, vol. 49, no. 7, pp. 976-982, 7. 2024. (https://doi.org/10.7840/kics.2024.49.7.976)
Vol. 49, No. 7 Index
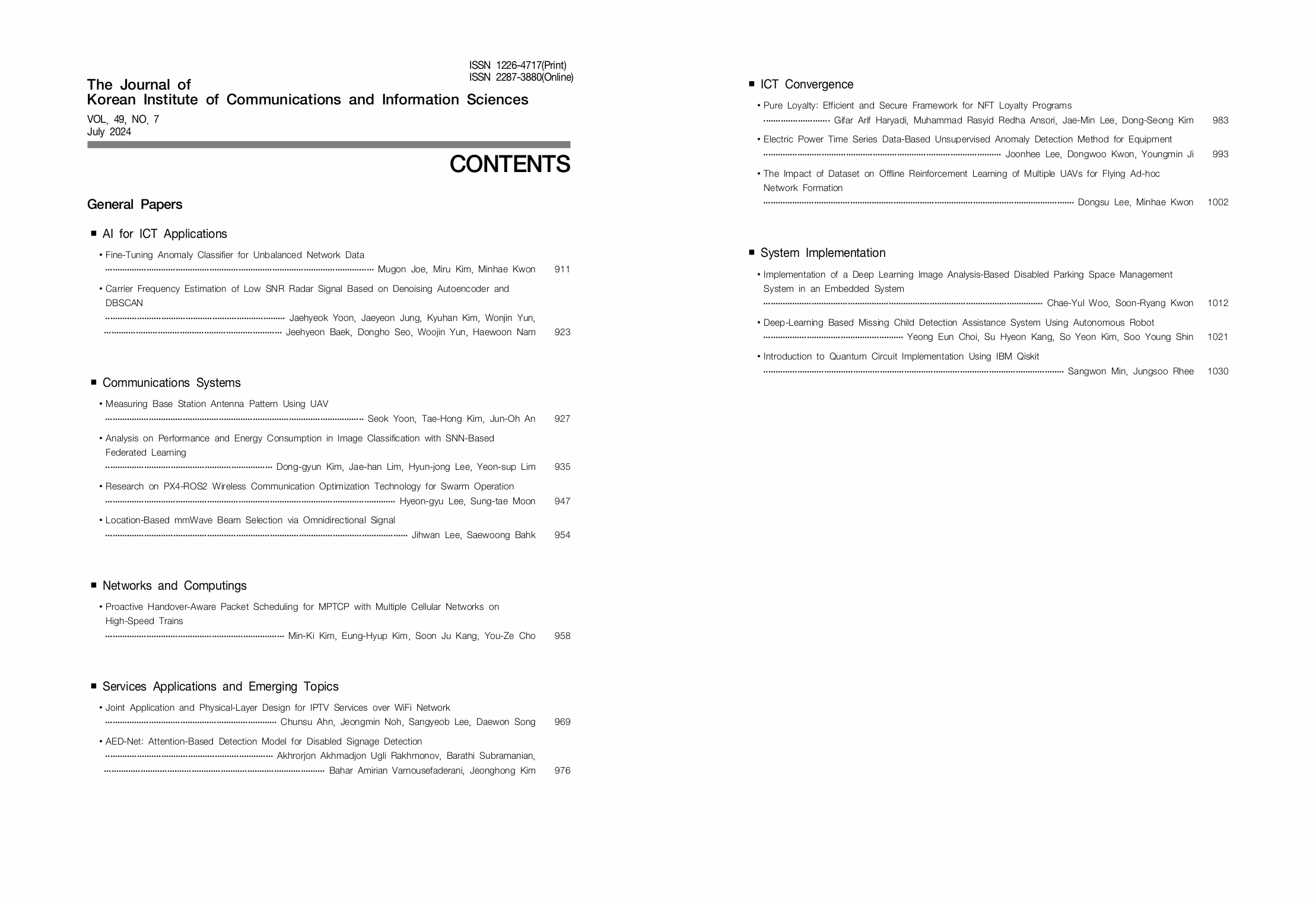