Electric Power Time Series Data-Based Unsupervised Anomaly Detection Method for Equipment
Vol. 49, No. 7, pp. 993-1001, Jul. 2024

PDF Full-Text
Abstract
Statistics
Cumulative Counts from November, 2022
Multiple requests among the same browser session are counted as one view. If you mouse over a chart, the values of data points will be shown.
Multiple requests among the same browser session are counted as one view. If you mouse over a chart, the values of data points will be shown.
|
Cite this article
[IEEE Style]
J. Lee, D. Kwon, Y. Ji, "Electric Power Time Series Data-Based Unsupervised Anomaly Detection Method for Equipment," The Journal of Korean Institute of Communications and Information Sciences, vol. 49, no. 7, pp. 993-1001, 2024. DOI: 10.7840/kics.2024.49.7.993.
[ACM Style]
Joonhee Lee, Dongwoo Kwon, and Youngmin Ji. 2024. Electric Power Time Series Data-Based Unsupervised Anomaly Detection Method for Equipment. The Journal of Korean Institute of Communications and Information Sciences, 49, 7, (2024), 993-1001. DOI: 10.7840/kics.2024.49.7.993.
[KICS Style]
Joonhee Lee, Dongwoo Kwon, Youngmin Ji, "Electric Power Time Series Data-Based Unsupervised Anomaly Detection Method for Equipment," The Journal of Korean Institute of Communications and Information Sciences, vol. 49, no. 7, pp. 993-1001, 7. 2024. (https://doi.org/10.7840/kics.2024.49.7.993)
Vol. 49, No. 7 Index
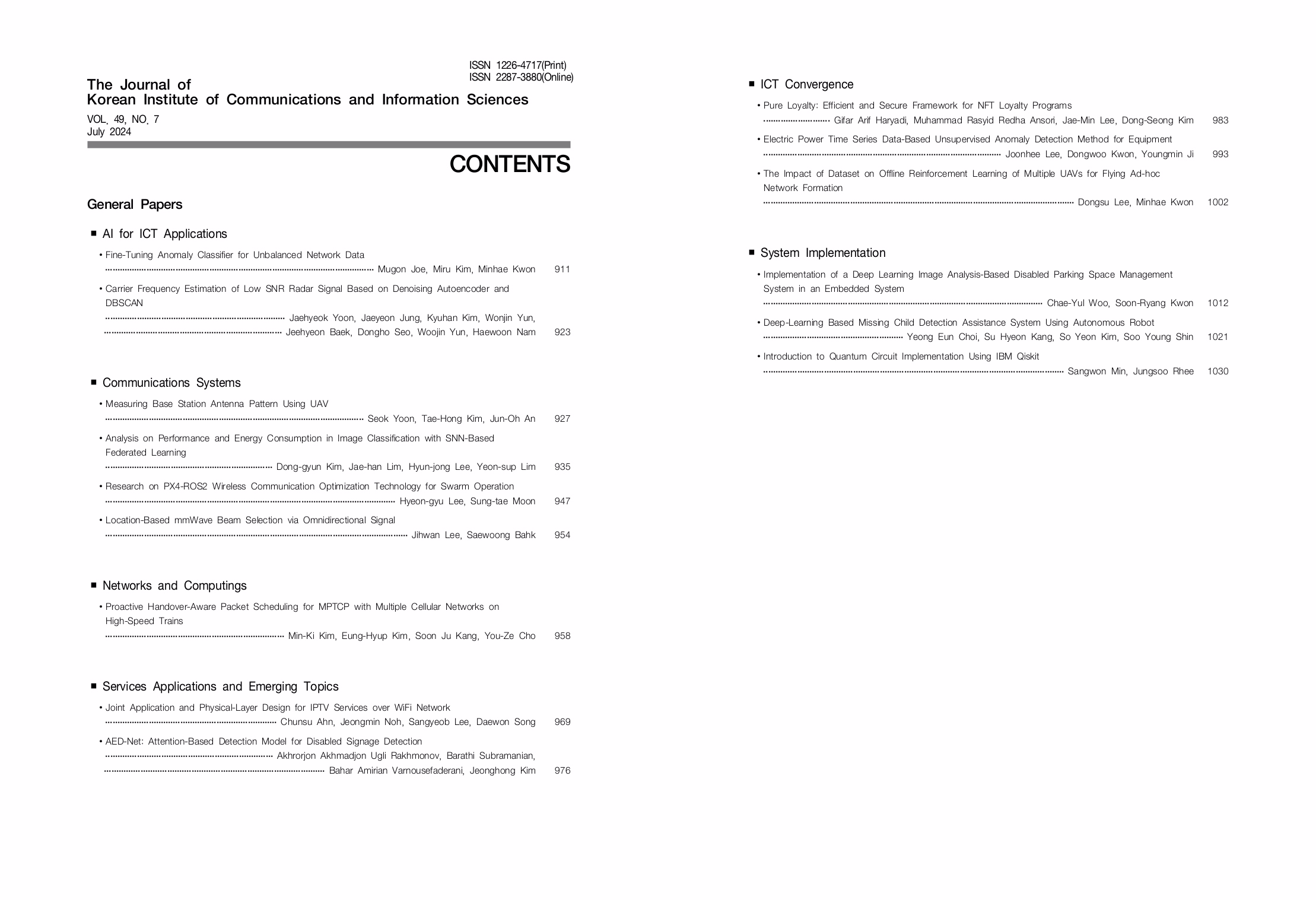