Multiple Access Control Protocol using Deep-Reinforcement Learning in Heterogeneous Wireless Networks
Vol. 49, No. 1, pp. 88-94, Jan. 2024

Abstract
Statistics
Cumulative Counts from November, 2022
Multiple requests among the same browser session are counted as one view. If you mouse over a chart, the values of data points will be shown.
Multiple requests among the same browser session are counted as one view. If you mouse over a chart, the values of data points will be shown.
|
Cite this article
[IEEE Style]
D. Kim and K. Shin, "Multiple Access Control Protocol using Deep-Reinforcement Learning in Heterogeneous Wireless Networks," The Journal of Korean Institute of Communications and Information Sciences, vol. 49, no. 1, pp. 88-94, 2024. DOI: 10.7840/kics.2024.49.1.88.
[ACM Style]
Do-won Kim and Kyung-seop Shin. 2024. Multiple Access Control Protocol using Deep-Reinforcement Learning in Heterogeneous Wireless Networks. The Journal of Korean Institute of Communications and Information Sciences, 49, 1, (2024), 88-94. DOI: 10.7840/kics.2024.49.1.88.
[KICS Style]
Do-won Kim and Kyung-seop Shin, "Multiple Access Control Protocol using Deep-Reinforcement Learning in Heterogeneous Wireless Networks," The Journal of Korean Institute of Communications and Information Sciences, vol. 49, no. 1, pp. 88-94, 1. 2024. (https://doi.org/10.7840/kics.2024.49.1.88)
Vol. 49, No. 1 Index
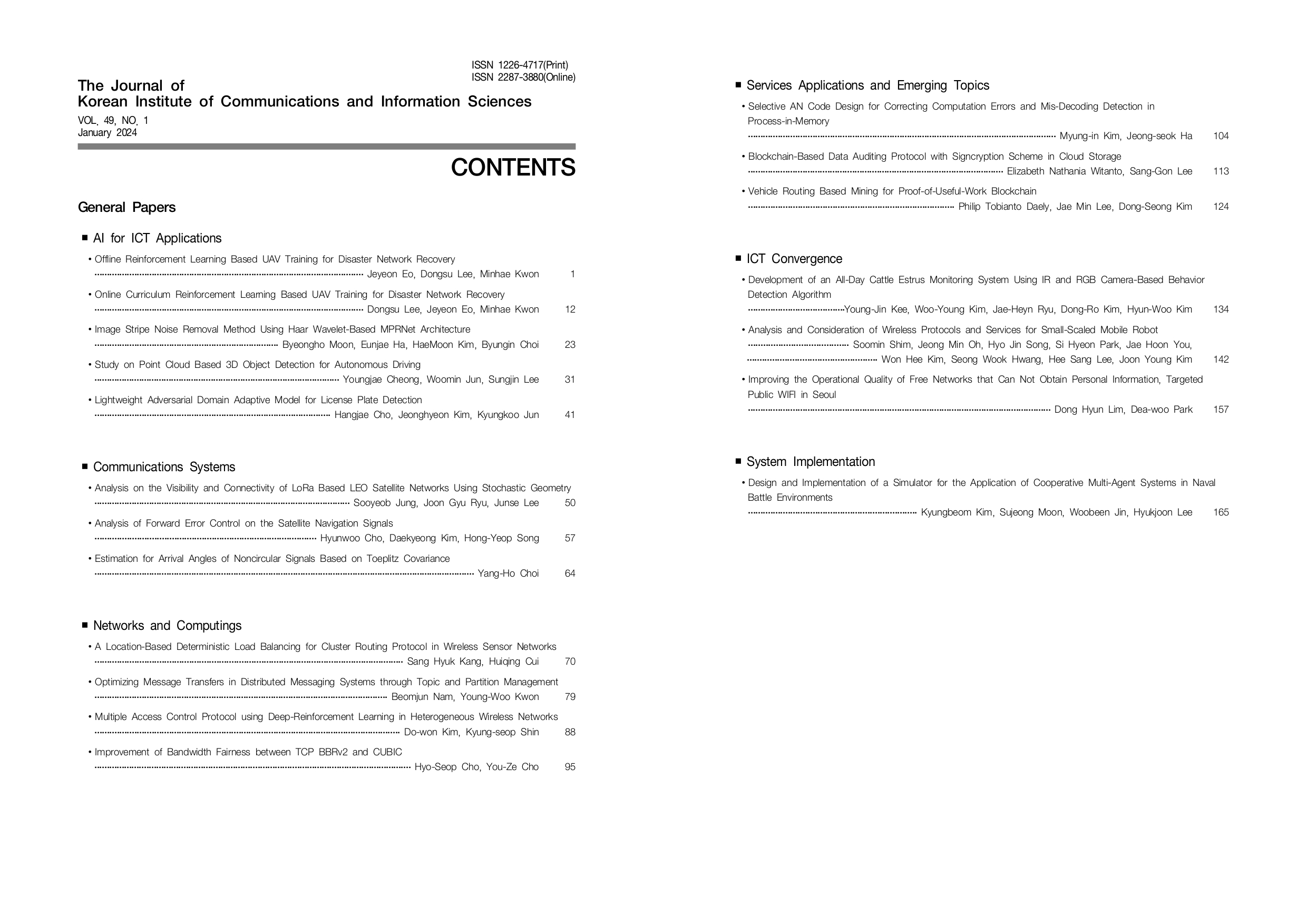