Deep Learning-Based Approaches for Nucleus Segmentation
Vol. 49, No. 4, pp. 620-629, Apr. 2024

PDF Full-Text
Abstract
Statistics
Cumulative Counts from November, 2022
Multiple requests among the same browser session are counted as one view. If you mouse over a chart, the values of data points will be shown.
Multiple requests among the same browser session are counted as one view. If you mouse over a chart, the values of data points will be shown.
|
Cite this article
[IEEE Style]
D. C. Bui and M. Yoo, "Deep Learning-Based Approaches for Nucleus Segmentation," The Journal of Korean Institute of Communications and Information Sciences, vol. 49, no. 4, pp. 620-629, 2024. DOI: 10.7840/kics.2024.49.4.620.
[ACM Style]
Duy Cuong Bui and Myungsik Yoo. 2024. Deep Learning-Based Approaches for Nucleus Segmentation. The Journal of Korean Institute of Communications and Information Sciences, 49, 4, (2024), 620-629. DOI: 10.7840/kics.2024.49.4.620.
[KICS Style]
Duy Cuong Bui and Myungsik Yoo, "Deep Learning-Based Approaches for Nucleus Segmentation," The Journal of Korean Institute of Communications and Information Sciences, vol. 49, no. 4, pp. 620-629, 4. 2024. (https://doi.org/10.7840/kics.2024.49.4.620)
Vol. 49, No. 4 Index
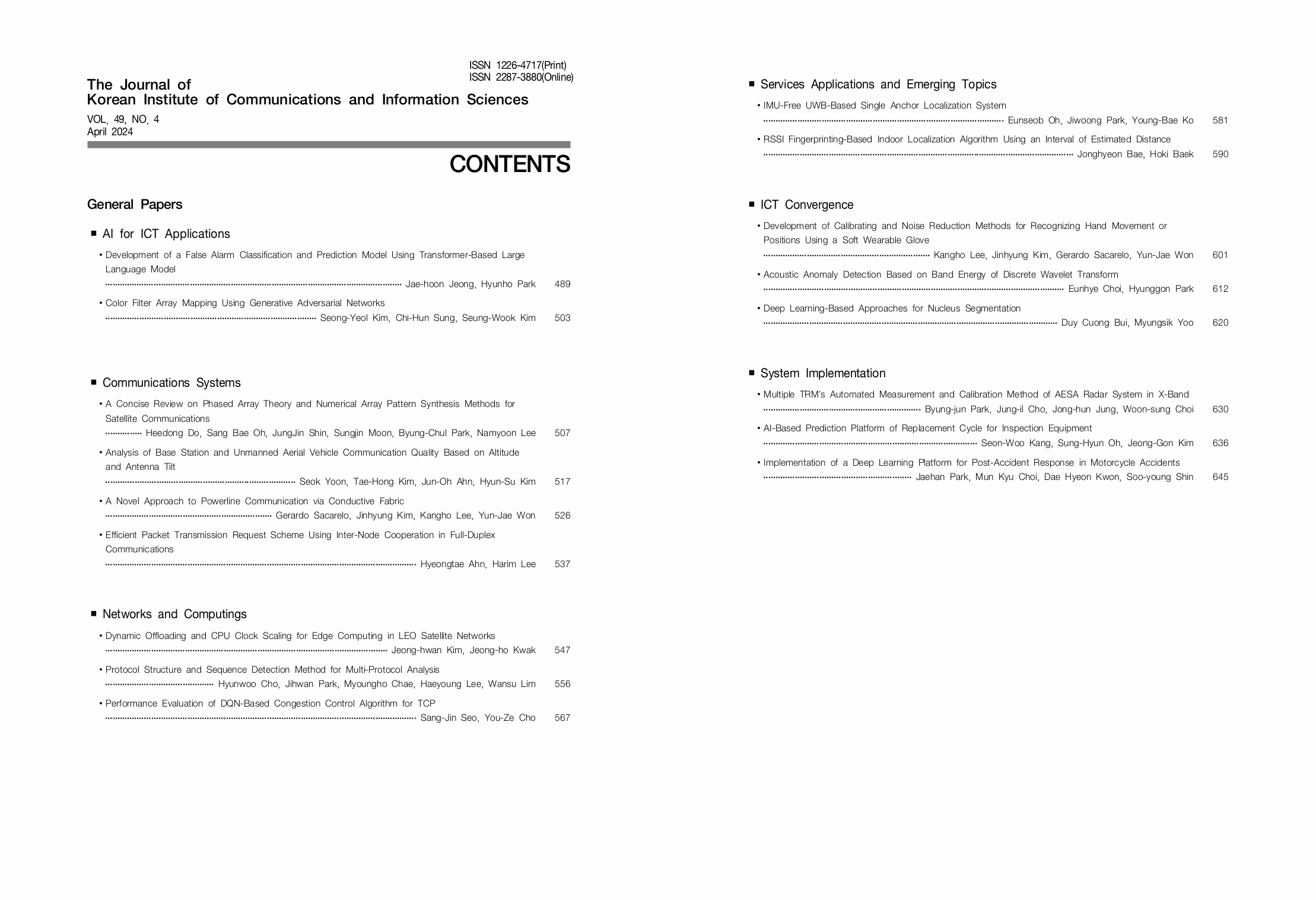