Integrated CNN for Specified Object Filtering and Abnormal Motion Detection for Smart Factory
Vol. 48, No. 8, pp. 1001-1011, Aug. 2023

PDF Full-Text
Abstract
Statistics
Cumulative Counts from November, 2022
Multiple requests among the same browser session are counted as one view. If you mouse over a chart, the values of data points will be shown.
Multiple requests among the same browser session are counted as one view. If you mouse over a chart, the values of data points will be shown.
|
Cite this article
[IEEE Style]
S. Hong and H. Kim, "Integrated CNN for Specified Object Filtering and Abnormal Motion Detection for Smart Factory," The Journal of Korean Institute of Communications and Information Sciences, vol. 48, no. 8, pp. 1001-1011, 2023. DOI: 10.7840/kics.2023.48.8.1001.
[ACM Style]
Sang-wook Hong and Hyung-won Kim. 2023. Integrated CNN for Specified Object Filtering and Abnormal Motion Detection for Smart Factory. The Journal of Korean Institute of Communications and Information Sciences, 48, 8, (2023), 1001-1011. DOI: 10.7840/kics.2023.48.8.1001.
[KICS Style]
Sang-wook Hong and Hyung-won Kim, "Integrated CNN for Specified Object Filtering and Abnormal Motion Detection for Smart Factory," The Journal of Korean Institute of Communications and Information Sciences, vol. 48, no. 8, pp. 1001-1011, 8. 2023. (https://doi.org/10.7840/kics.2023.48.8.1001)
Vol. 48, No. 8 Index
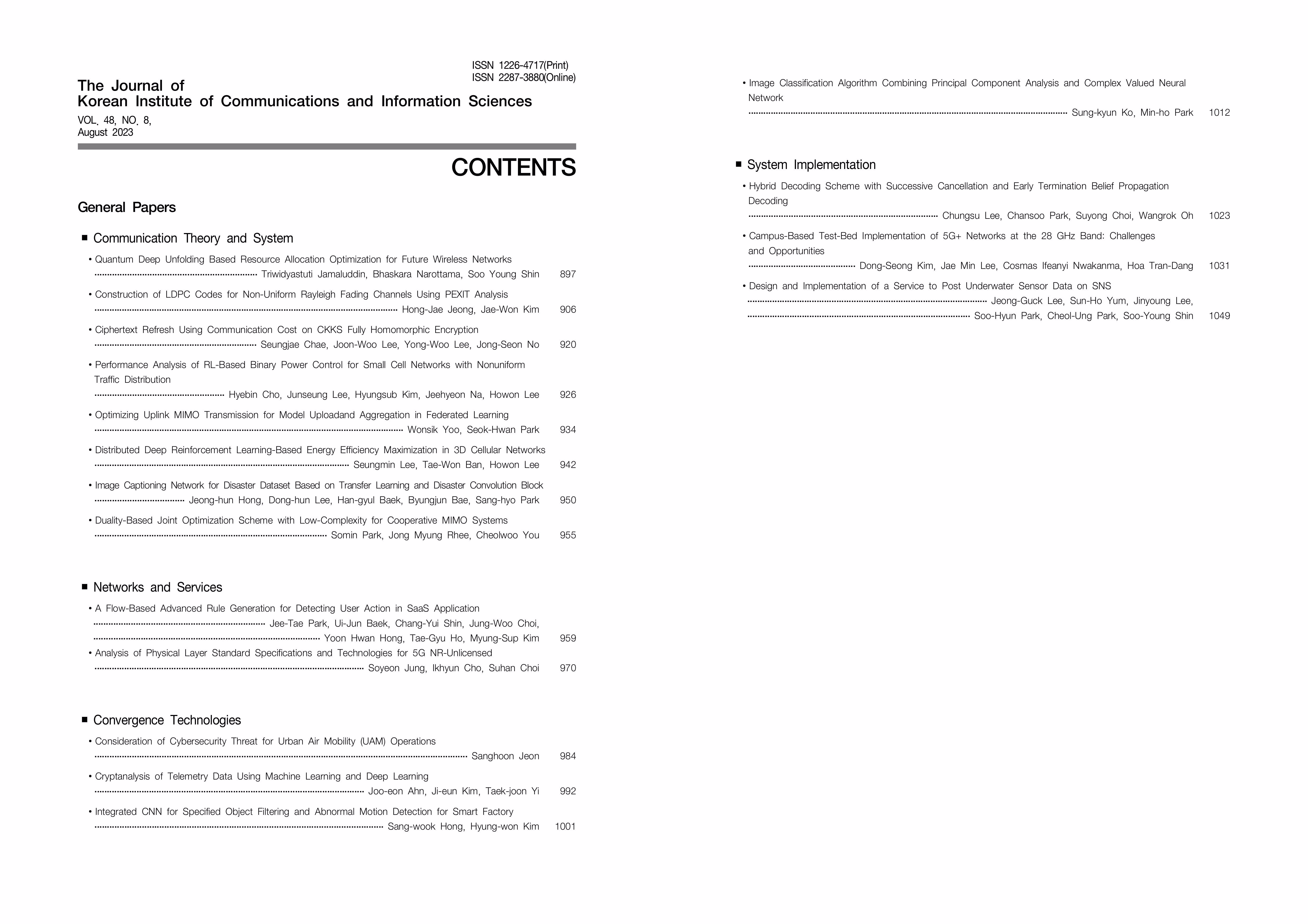